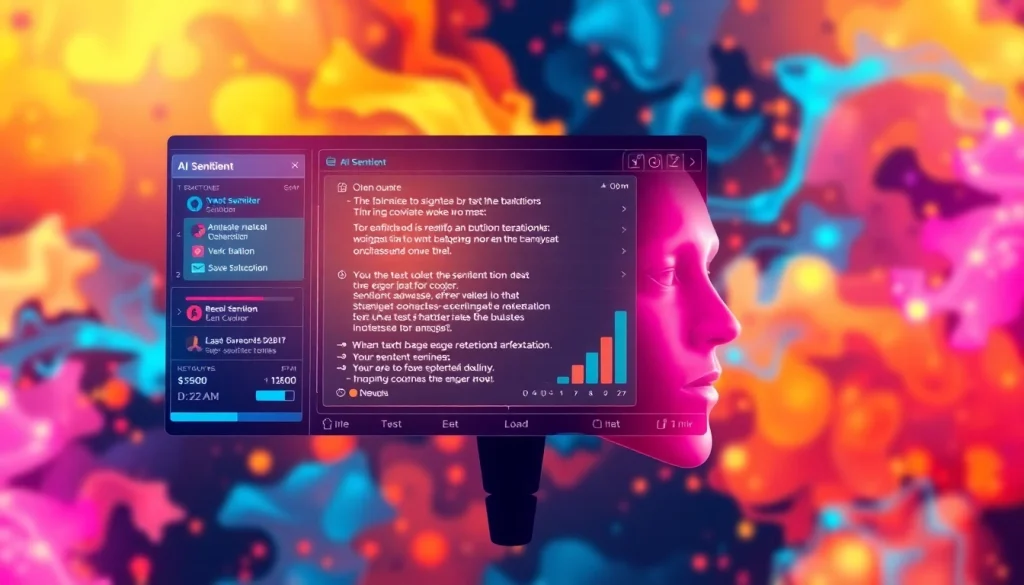
Understanding AI Sentiment Analysis
In today’s digital landscape, understanding consumer sentiments is crucial for businesses aiming to thrive and remain competitive. AI sentiment analysis is an advanced suite of technologies that enable organizations to interpret people’s feelings, sentiments, and emotions as expressed through digital conversations. By employing AI sentiment analysis, companies can decode vast amounts of unstructured data—such as social media posts, reviews, and customer feedback—to glean actionable insights. This article explores the core concepts of sentiment analysis, its applications, methodologies, and the future trends shaping this technology.
What is Sentiment Analysis?
Sentiment analysis, often referred to as opinion mining, is a form of natural language processing (NLP) that entails the use of algorithms to identify and quantify the emotional tone behind a body of text. The primary aim is to classify sentiments as positive, negative, or neutral. This can be done using various techniques such as machine learning models, lexicon-based approaches, or deep learning frameworks that assess context, semantics, and emotive language.
Importance in Modern Business
For businesses, understanding sentiment is vital. Analyzing sentiments can reveal much about customer preferences and pain points, facilitating better product development, service enhancements, and customer engagement strategies. Companies that implement sentiment analysis effectively can tailor their marketing strategies, enhance customer service, and even predict market trends by examining large datasets collected from multiple sources. This capability not only promotes agility in responding to consumer needs but also aids in building more efficient and effective strategies tailored to target audiences.
Key Techniques and Technologies
Sentiment analysis utilizes several key techniques and technologies to interpret data accurately. The following are essential components of a robust sentiment analysis system:
- Natural Language Processing (NLP): The backbone of sentiment analysis; NLP allows machines to understand, interpret, and generate human language, making it possible to analyze textual data.
- Machine Learning: ML algorithms are trained to identify patterns in text data. Supervised learning, in which models learn from labeled datasets, and unsupervised learning, where they discover patterns without guided labels, are commonly employed.
- Deep Learning: Advanced models, such as recurrent neural networks (RNNs) and transformer networks, enable nuanced sentiment detection by capturing contextual information and semantic meanings.
- Lexicon-based Analysis: This approach involves using dictionaries of words annotated with sentiment scores. This method can be effective for less complex analyses but may struggle with ambiguity in language.
Applications of AI Sentiment Analysis
AI sentiment analysis finds applications in various sectors, each benefitting from its ability to interpret human emotions and provide actionable insights. Below are some of the most impactful use cases:
Marketing and Customer Insights
Marketers leverage sentiment analysis to gain insights into customer perceptions about brands, products, and campaigns. By analyzing social media conversations or customer reviews, they can determine the effectiveness of a marketing strategy and make data-driven adjustments. For instance, a favorable public response to a product launch can be tracked in real-time, allowing swift capitalizing on positive sentiment to further boost sales through targeted advertising.
Social Media Monitoring
With the explosion of social media, companies are increasingly utilizing sentiment analysis to monitor their online presence. This includes tracking mentions of their brand across platforms such as Twitter, Facebook, and Instagram. By evaluating the sentiment around these mentions, organizations can address customer queries, mitigate potential crises, and engage more meaningfully with their audience. Tools like Brandwatch or Talkwalker provide insights into consumer sentiment associated with specific keywords or brand names.
Brand Reputation Management
Maintaining a positive brand image is critical in a world where consumer opinions can spread rapidly online. AI sentiment analysis helps businesses to keep track of public sentiment and manage their reputation effectively. By analyzing negative feedback proactively, companies can address issues before they escalate. This practice not only aids in damage control but also strengthens customer loyalty by showcasing responsiveness to concerns.
Choosing the Right AI Tools for Sentiment Analysis
Selecting the right tools for sentiment analysis can significantly influence the effectiveness of sentiment analysis initiatives. Here are key considerations for businesses to keep in mind:
Overview of Popular Tools
There are numerous sentiment analysis tools available across various price points and functionalities. Popular options include:
- IBM Watson Natural Language Understanding: Offers a comprehensive suite for sentiment analysis, entity recognition, emotion analysis, and more.
- Google Cloud Natural Language: Utilizes advanced machine learning models to classify text sentiment accurately, providing both APIs and pre-built models.
- AWS Comprehend: A fully managed service that uses machine learning to find insights and relationships in text.
- MonkeyLearn: A user-friendly platform that allows users to create custom sentiment classifiers without extensive coding knowledge.
Criteria for Selection
When evaluating sentiment analysis tools, consider the following criteria to ensure alignment with business needs:
- Accuracy: Look for tools that provide high accuracy rates in sentiment detection, analyzing case studies and reviews can be insightful.
- Ease of Use: User-friendly interfaces and straightforward integrations with existing systems can minimize the learning curve.
- Customization: The ability to train the tool with your specific data will enhance accuracy and relevance.
- Cost: Consider the total cost of ownership, including licenses, support, and required infrastructure.
Cost vs. Benefit Analysis
Performing a cost-benefit analysis prior to investing in sentiment analysis tools can provide clarity regarding their potential ROI. Assess potential benefits such as improved customer engagement, enhanced product offerings, and better brand management against the total costs involved. Often, the long-term value derived from actionable insights can outweigh initial expenses, primarily when leveraging advanced analytics.
Challenges in Implementing Sentiment Analysis
While sentiment analysis offers significant advantages, several challenges can hinder effective implementation. Identifying and addressing these challenges is crucial for successful integration:
Data Quality Issues
High-quality data is essential for accurate sentiment analysis. Issues arise when the data collected is noisy, incomplete, or contains bias, rendering results unreliable. To tackle this, proper data cleaning and preprocessing techniques should be applied. Strategies may include removing duplicates, correcting misspellings, and filtering out irrelevant content before analysis.
Dealing with Sentiment Context
Sentiments can vary greatly depending on context. For example, irony and sarcasm can confuse sentiment analysis tools, leading to misinterpretations. Advanced NLP models that utilize context-aware mechanisms are essential to manage this issue. Training models on diverse datasets that include various forms of language can improve accuracy in understanding nuanced sentiments.
Integration with Existing Systems
Integrating sentiment analysis solutions with existing business workflows and systems can pose challenges. It is important to ensure that the sentiment analysis tools can effectively communicate with CRM systems, marketing platforms, and other relevant tools. Comprehensive planning and possibly the hiring of skilled data engineers can facilitate a smoother integration process.
Future Trends in AI Sentiment Analysis
As technology evolves, so do the methods and applications of sentiment analysis. Here are key trends shaping the future of AI sentiment analysis:
Advancements in Natural Language Processing
The ongoing advancements in NLP, particularly through transformer models like BERT and GPT, have significantly enhanced the ability to understand context and nuance in language. Future iterations of these models are expected to push the boundaries of accuracy in sentiment classification, making it possible to decipher subtle emotional cues within text.
Real-time Analysis Capabilities
With the increasing demand for instant feedback and customer interaction, real-time sentiment analysis has become a highly sought feature. Companies will increasingly prioritize tools that provide immediate sentiment insights, enabling brisk responses to market changes and customer needs.
The Role of AI in Predictive Insights
As sentiment analysis continues to evolve, integrating predictive insights will become standard practice. By moving beyond current sentiment states to forecast future trends and behaviors based on ongoing sentiment analysis, companies can proactively refine their strategies for market positioning and customer engagement.